In the past decade, The world of AI has undergone a major change, just as a strange new type of intelligence has appeared on the planet. The capabilities and weaknesses of this AI remain mysterious, and we still understand very little.
The Evolution of Human Intelligence and AI
To understand AI in depth It is necessary to look back at the historical context of biological intelligence. Starting with the common ancestor of all vertebrates, which is about 500 million years old. From this small creature Evolution has created a more complex brain, so that humans have been able to invent deep mathematics and physics to understand the universe, from quarks to cosmology, in a matter of a few hundred years. Without relying on AI like ChatGPT
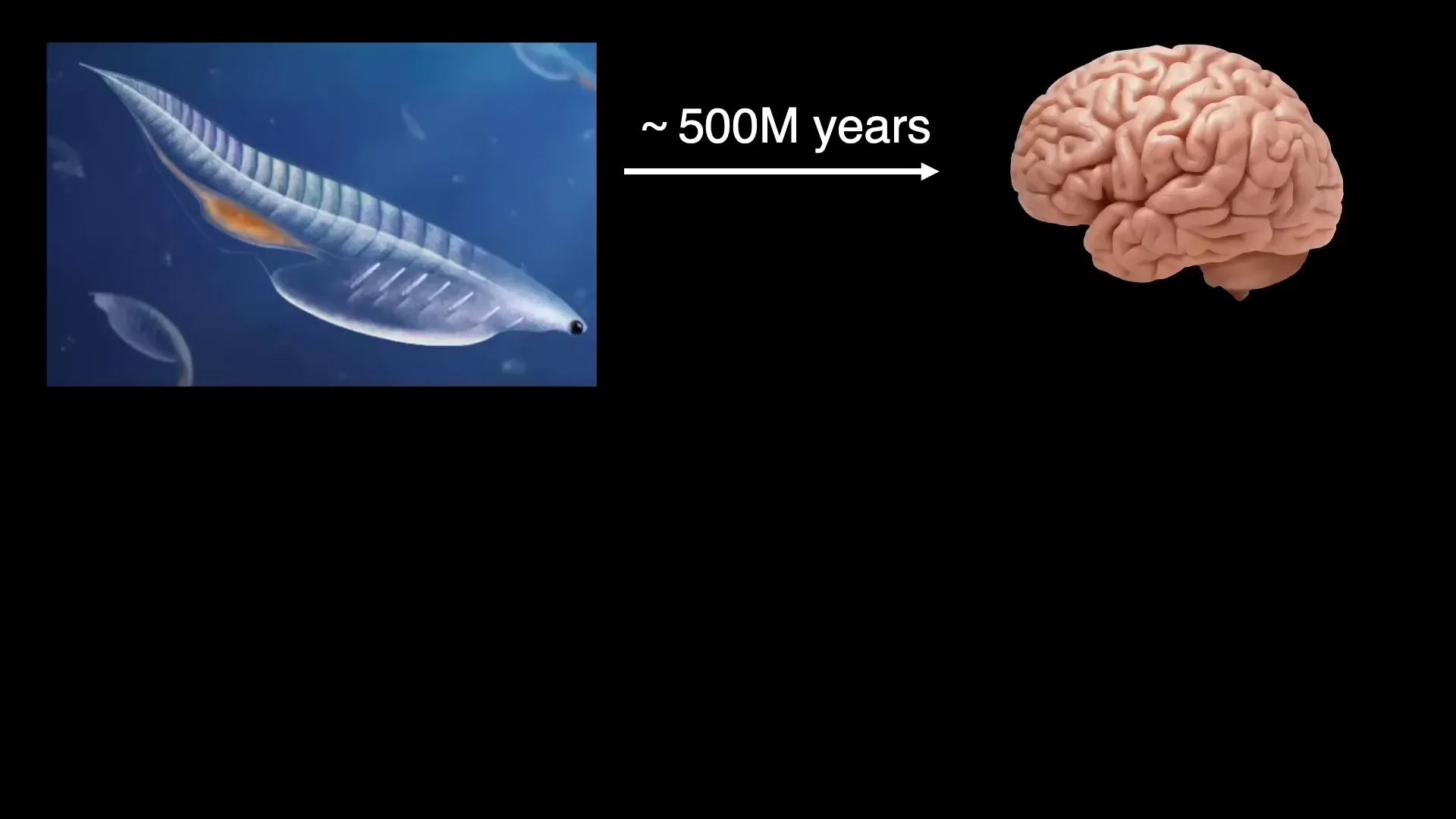
The development of AI in the last decade has been remarkable. We must combine knowledge from physics, mathematics, neuroscience, psychology and computer science to create a new science called " Science of Intelligence "That will help us understand both biological intelligence and create better AI.
1. Solving the problem of large data requirements (Data Efficiency)
Today's AI requires a huge amount of data to train, for example, large language models need to be trained with up to a trillion words. Humans only get about 100 million words of information from actual learning, which is much less. Reading AI data trains takes up to 24,000 years by human standards.
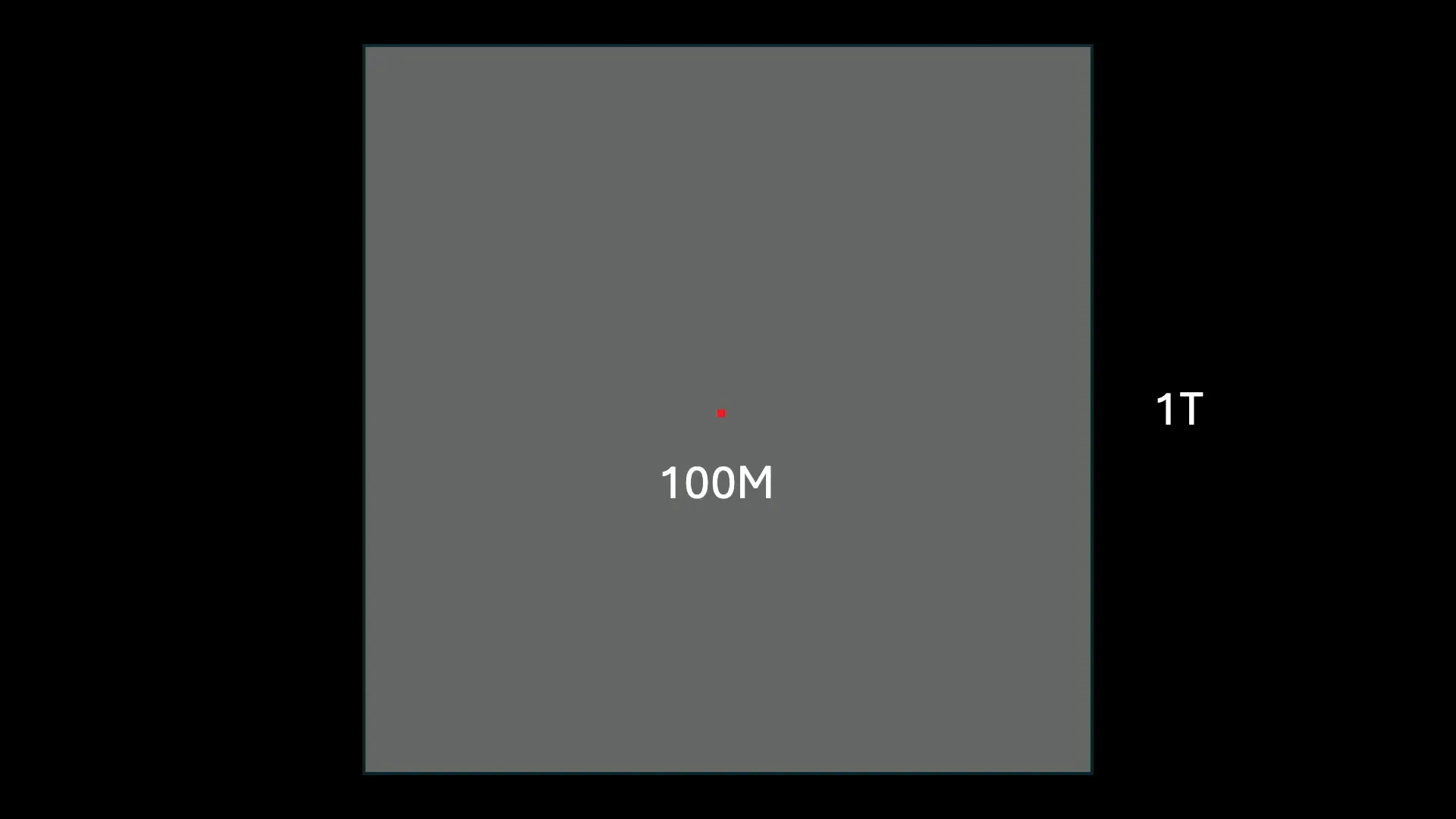
Despite the evolution of the brain over 500 million years, the data we receive through DNA is only about 700 megabytes, or the equivalent of about 600 million words.
Another problem that arises is the "Scaling Laws" or data size rules, which indicate that when more data is trending, the data will be more trending. Model errors are reduced at too slow a rate. That is, the data must be increased tenfold so that the error is reduced slightly, which is not sustainable in the long run.
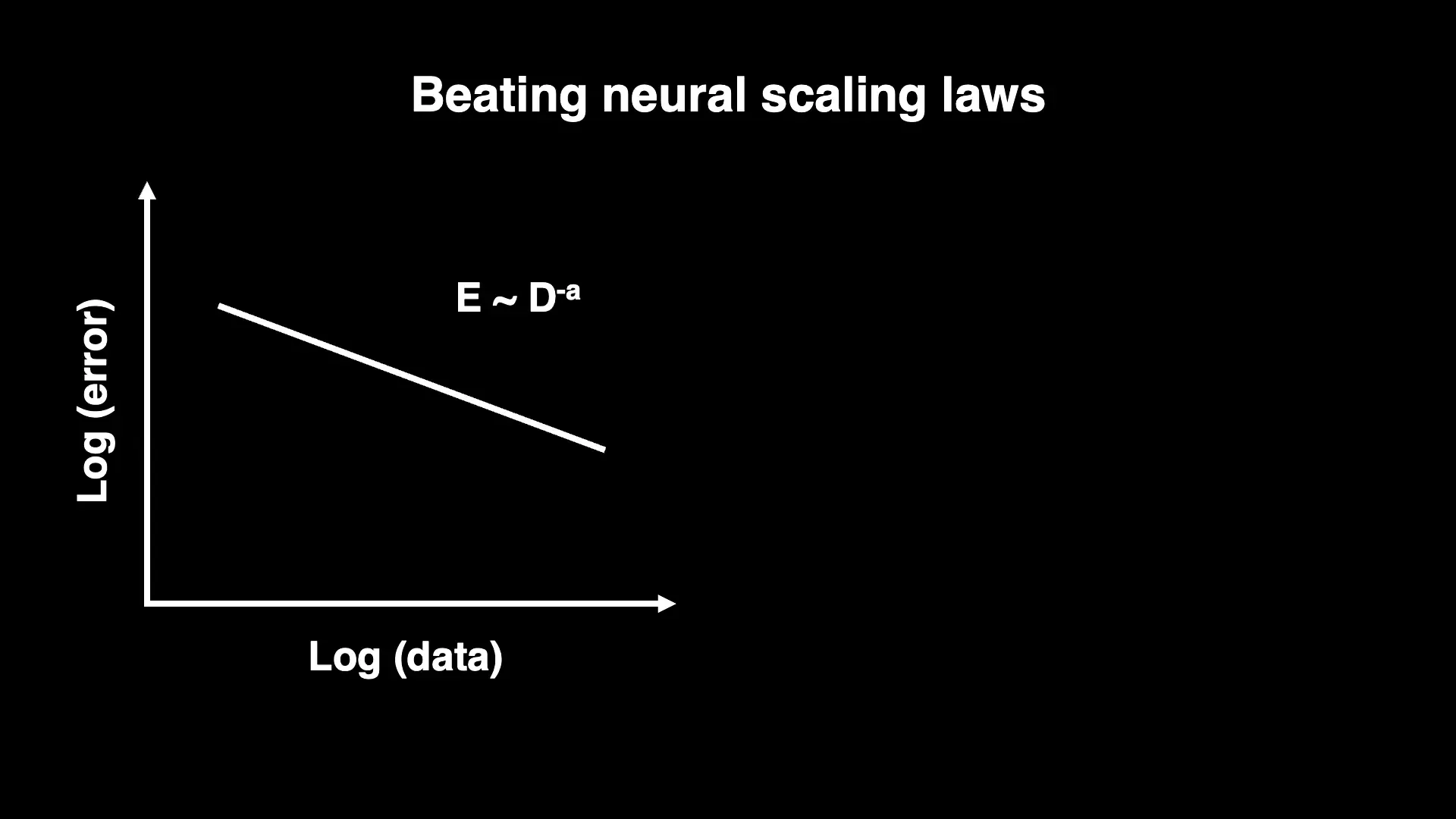
The team has developed a theory that explains why so much data is redundant and doesn't generate much new knowledge. This makes adding data not worth it. however If we can create a dataset that is free of duplication, selecting specific data with new material, it will help learning much more efficiently and can reduce more errors without adding a huge amount of data.
Example comparing AI training with teaching children
Imagine teaching a child to predict the next word in a random internet message all the time. We teach children in different ways, such as teaching math, we teach how to solve problems using steps (algorithms), so children can solve new problems with much less information. This is the way AI should evolve — from random learning to effective teaching, also known as " Machine Teaching "
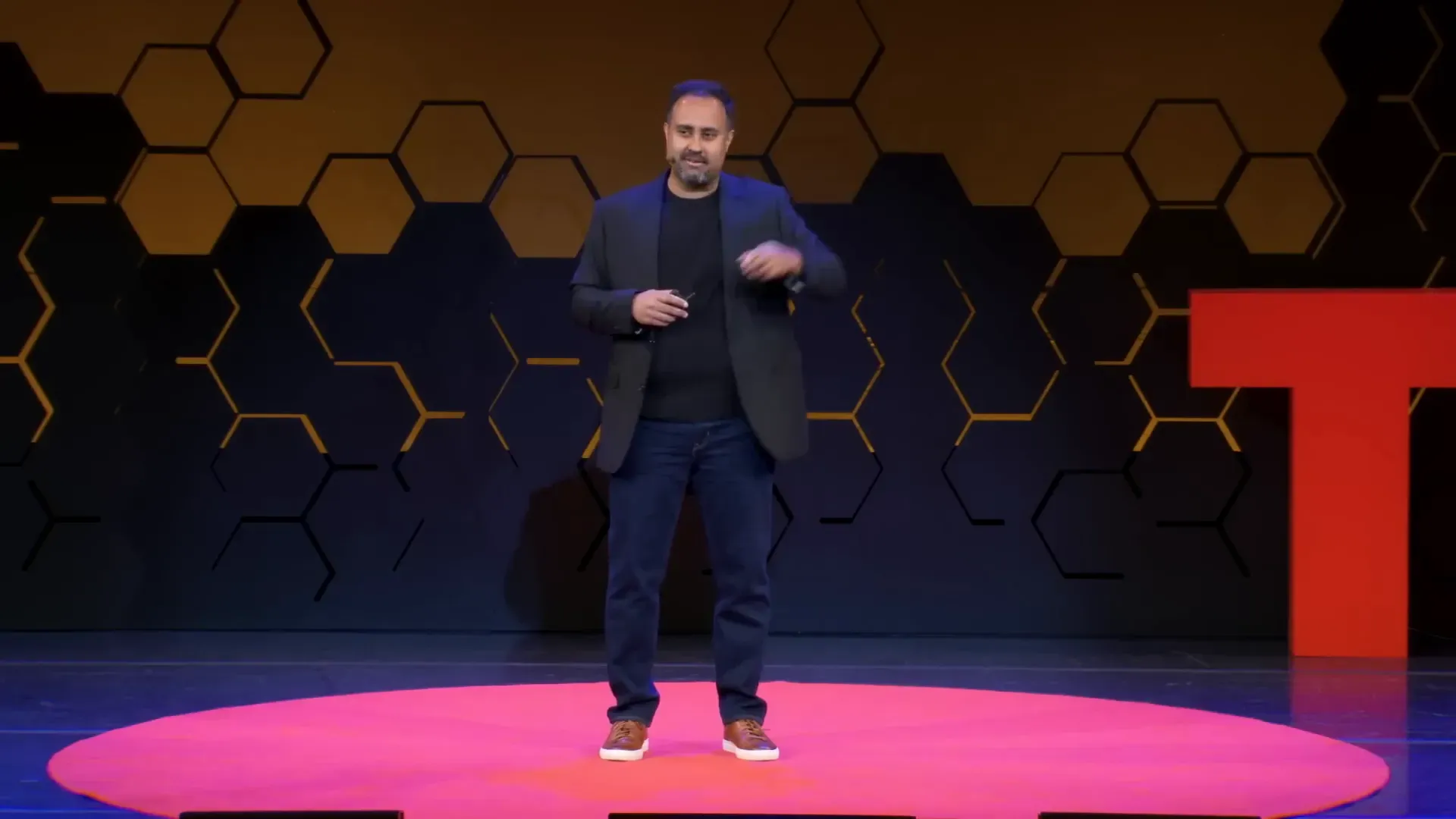
2. Energy Efficiency
The human brain only consumes 20 watts of power, compared to the old 100-watt light bulbs, but AI to train large models sometimes requires up to 10 million watts of power, or even talks of using nuclear power for a billion-watt data center.
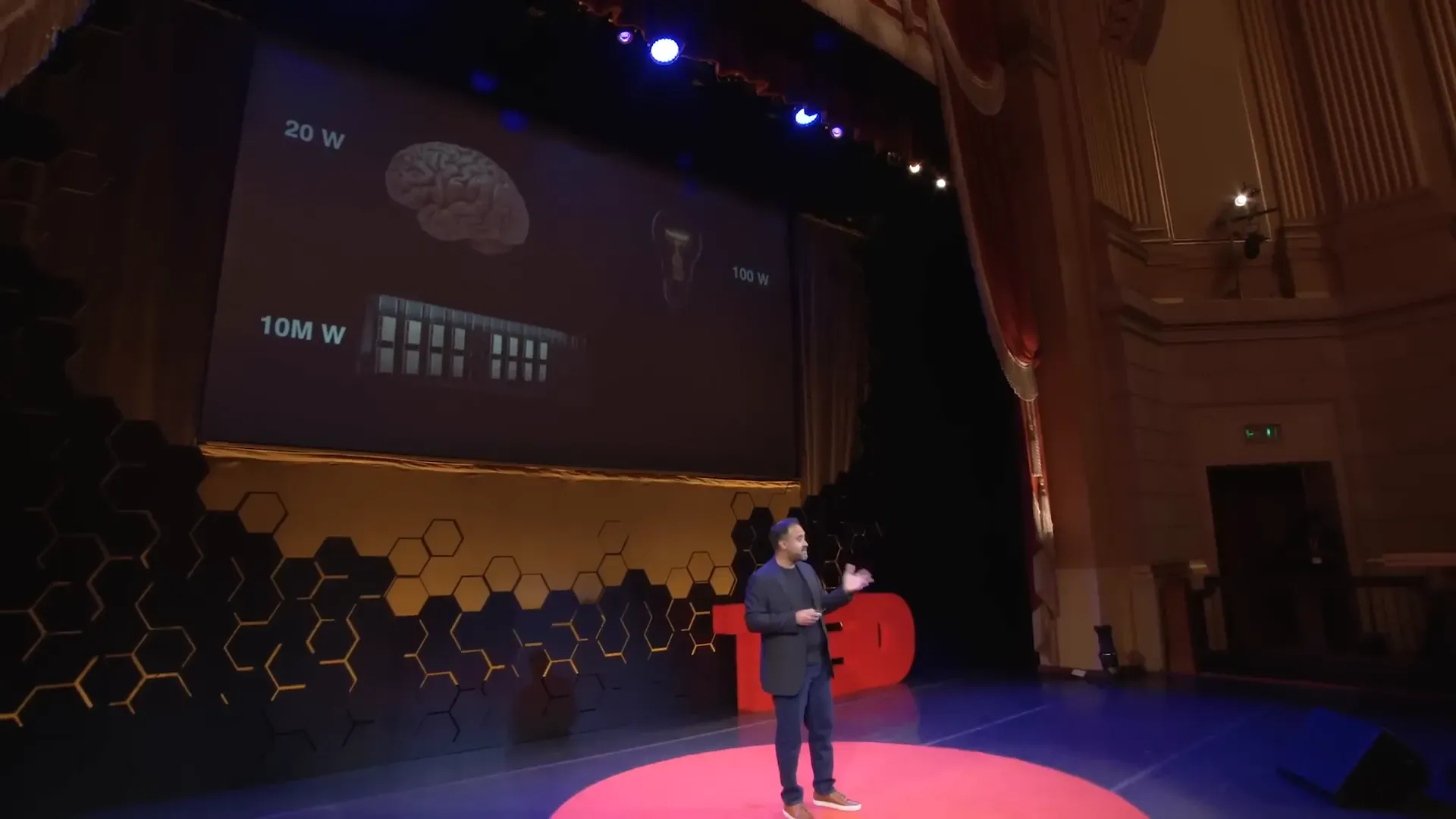
The reason AI consumes so much more energy than the human brain comes from the use of digital computers that require bit state changes. (bit flip) quickly and accurately. Fast and accurate state changes require high power. While the brain works differently. It uses slow and inaccurate calculations at each step, but only correctly calculates at the right time.
In addition, the brain uses principles that are directly compatible with physics, such as the positive in the brain is formed by the combination of neuronal voltages, which corresponds to Maxwell's law of electromagnetism. Computers use more complex and power-efficient transistor circuits.
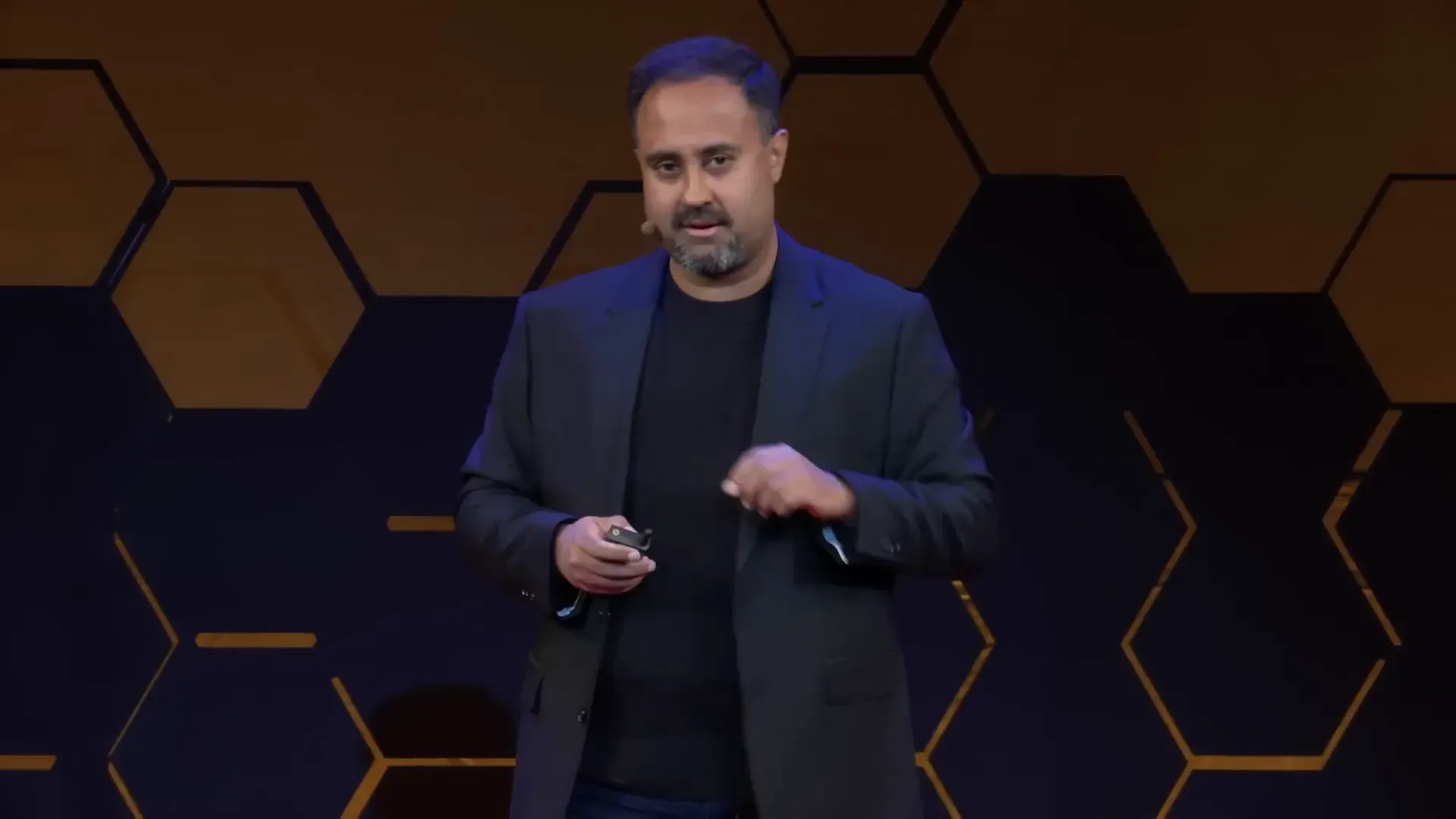
Therefore, creating energy-efficient AI must start from designing technologies from the electron level to new algorithms that are more compatible with the physics and dynamics of the system.
An interesting example is the calculation of sensing, which is the function of neurons. How much energy can we calculate the fundamental limit of the accuracy of perception consumption? And it was found that neurons use molecules similar to G-protein coupled receptors, which work close to this limit. This shows that biology can reach maximum efficiency based on the limitations of physics.
3. Leapfrogging Evolution with Quantum Neuromorphic Computing
Although AI learns from the brain and evolves, we can push the limits of evolution by incorporating brain algorithms with quantum hardware, such as using the electronic state of atoms instead of firing neurons, and photons instead of synapses.
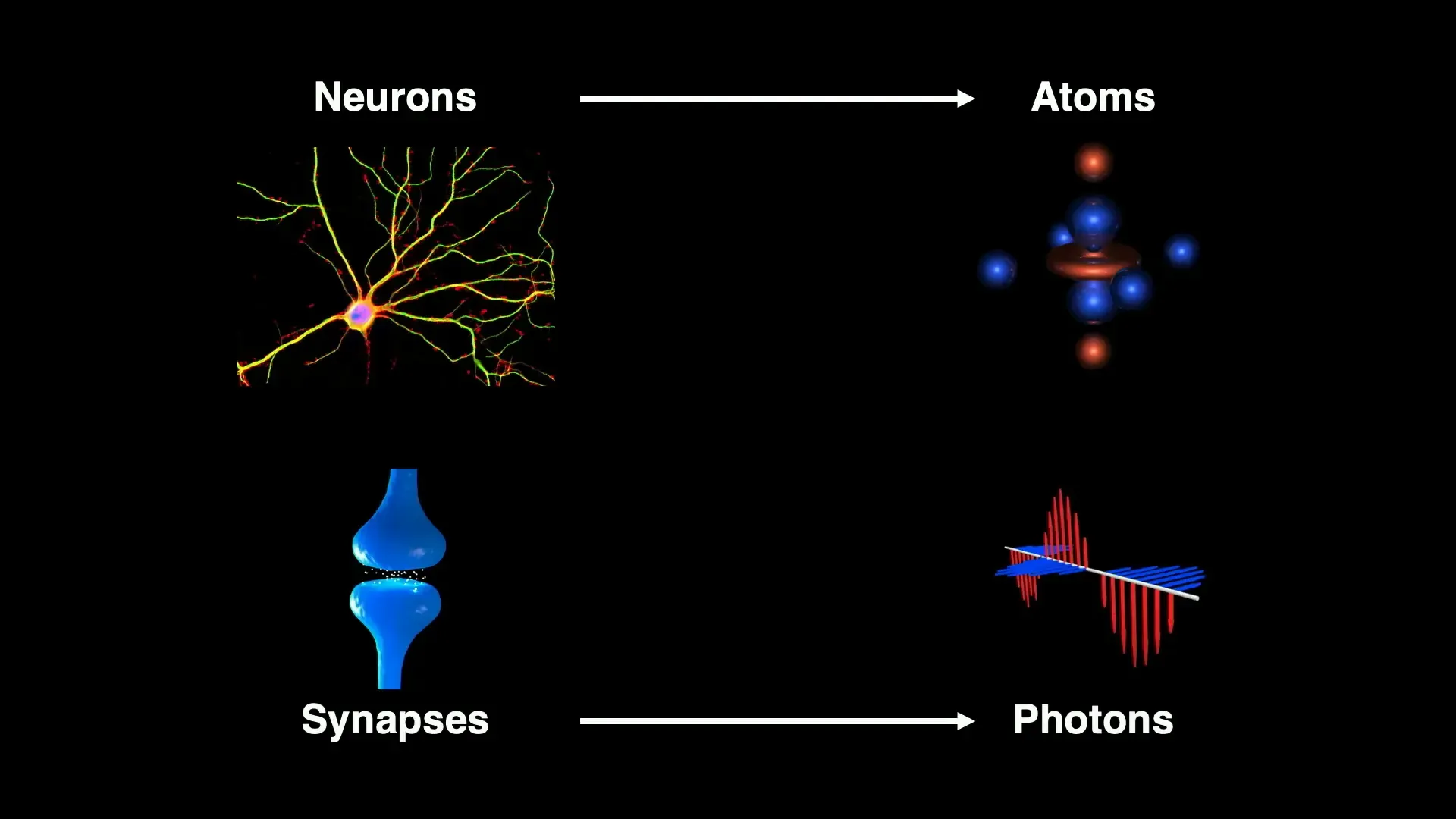
Creating associative memory with atoms and photons can now better increase the capacity, durability, and accuracy of data retrieval, and quantum optimizers that solve optimization problems in new ways can be created directly with photons.
This combination gave rise to a new branch called Quantum Neuromorphic Computing This is a new step for AI in the future.
4. Explainable AI and Understanding the Brain
AI allows for the creation of complex and highly accurate brain models, such as Retina's, which can accurately replicate the results of experiments over a period of more than two decades. This allows us to Digital Twin " or a digital model of the retina.
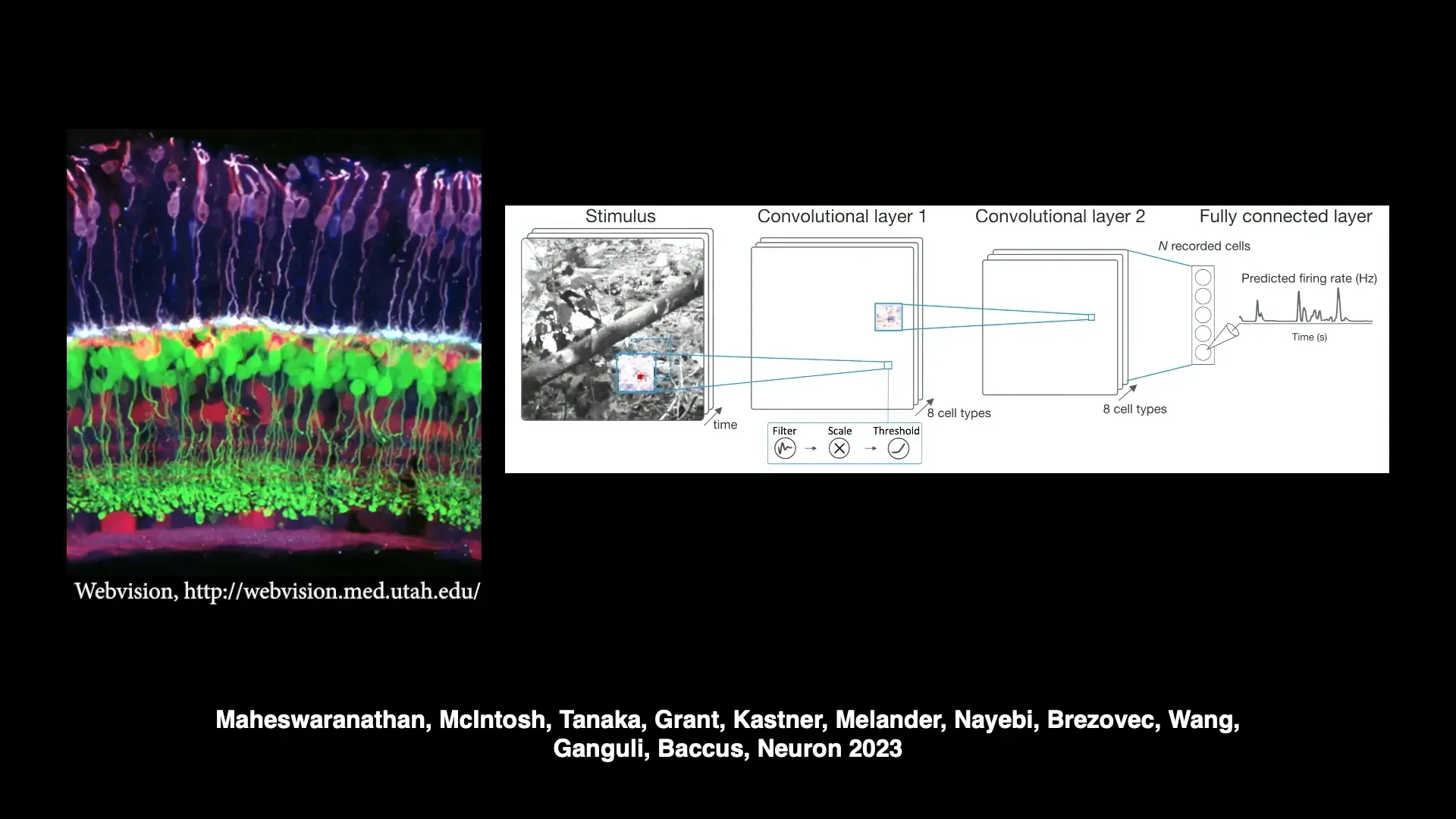
But the key question is, how does this model work? For example, in a simple experiment, track the movement of an unnaturally changing hand, which violates Newton's First Law of Motion. The model was able to accurately replicate the response of neurons in the retina that responded to this violation.
We develop techniques Explainable AI To extract the subcircuits of the model responsible for this behavior and explain its mechanism of operation. This is a major step forward in accelerating AI-based neuroscience discoveries.
5. Melding Minds and Machines
with Digital Twin s of the brain We can establish two-way communication between the brain and machines. By recording neural activity and modeling it, then using the theory of control ( Control Theory ) to learn the activity patterns that can control the model and write the same patterns back into the real brain to control the brain directly.
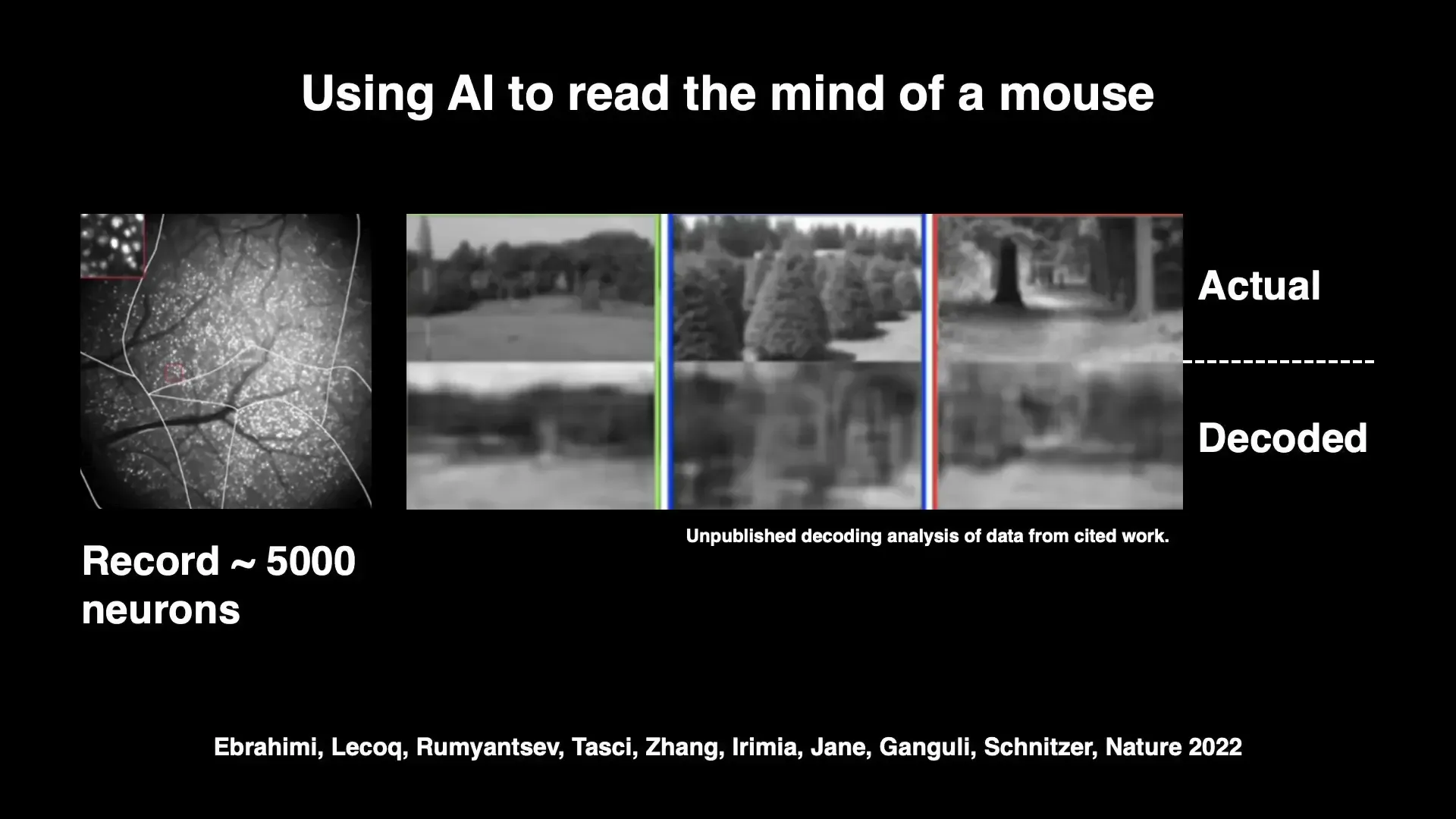
An interesting example is An experiment with mice that was able to decipher the images that the mice saw from brain activity, and was able to write neural activity to make the mice see the images that we determine, controlling only 20 neurons.
This opens the door to the possibility of understanding. Preserve and enhance human brain function in the future without limits.
Conclusion from Insiderly
The development of AI in the last decade has shown the emergence of a new type of intelligence that still has many mysteries and weaknesses. Understanding and Developing Effective and Understandable Artificial Intelligence It requires a new science that combines knowledge from many fields, including physics, neuroscience, and psychology.
Solving the problem of AI's massive data and high-power requirements will lead to the creation of more efficient and sustainable technologies, such as the creation of datasets without redundancy. The design of systems that fit with natural physics and the use of quantum computing combined with brain algorithms
in addition Explainable AI It will help us better understand the human brain, and establishing two-way communication between the brain and machines will be key to the development of medicine and technology in the future.
These challenges and opportunities require open and long-term work in academia, which will allow the world to benefit from real knowledge and innovation in the field of artificial intelligence and intelligence.
Technical Terminology (Jargon)
- Scaling Laws: A mathematical rule that says how model error decreases when increasing the amount of training data.
- Machine Teaching: AI teaching concepts by selecting useful and specific data instead of training with large amounts of random data.
- Quantum Neuromorphic Computing: Combining neurological algorithms with quantum hardware to create high-performance computer systems.
- Digital Twin: Digital models of real systems, such as the brain or retina, used for studies and experiments.
- Control Theory: A mathematical theory used to control the behavior of a system by adjusting the feedback values.