Language models are at the heart of AI that allow the system to predict the next word in a sentence from a received message. These models are developed to understand and generate contextually appropriate messages. They are trained with a huge amount of information from the Internet, books, and public sources to be well-rounded and able to respond to questions or instructions received accurately.
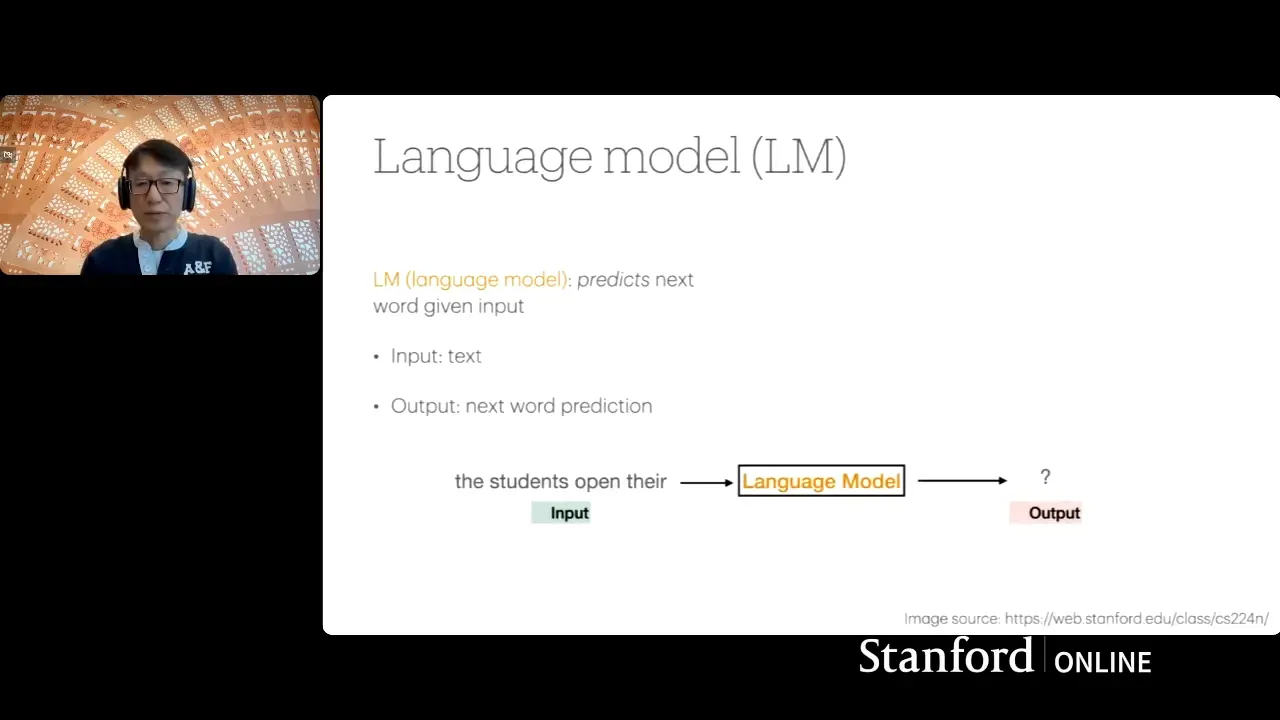
Language Model Training: Pre-Training and Post-Training Steps
Language model training is divided into two main stages:
- Pre-Training: The model is trained with a large amount of data to learn to predict the next word in the text (Next Token Prediction) using unsupervised learning techniques, giving the model extensive knowledge of language and meaning.
- Post-Training: After Pre-Training, the model is further customized to better respond to commands or questions. The training uses specific structured data, such as appropriate command and answer datasets, and also uses the Reinforcement Learning with Human Feedback (RLHF) technique to allow the model to learn from human preferences and provide more useful responses.
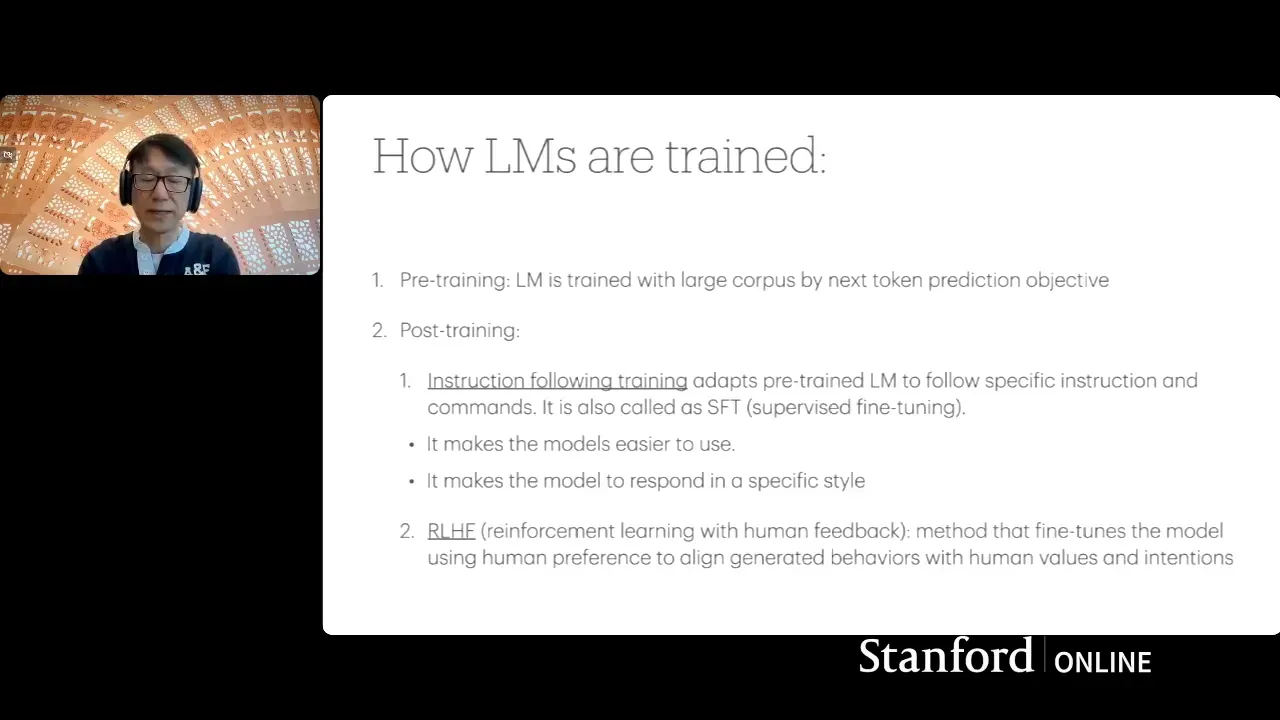
Language Model Implementation and Prompting
Language models typically take input data as freeform text and generate responses based on commands or questions received. It is very important to prepare a prompt so that the model clearly understands the needs and responds correctly.
A good Prompt preparation principle should include:
- Write the instructions clearly and in detail so that the model understands what it needs well.
- Include few-shot examples to show the style and style of the desired answer.
- Provide additional information or context to help reduce the problem of creating information errors (Hallucination).
- Give the model time to think and analyze using the Chain of Thought (COT) technique to allow the model to create a thinking process before giving an answer.
- Break down complex tasks into sub-steps, so that the model works sequentially one at a time.
- Track and record results systematically to help improve and analyze work.
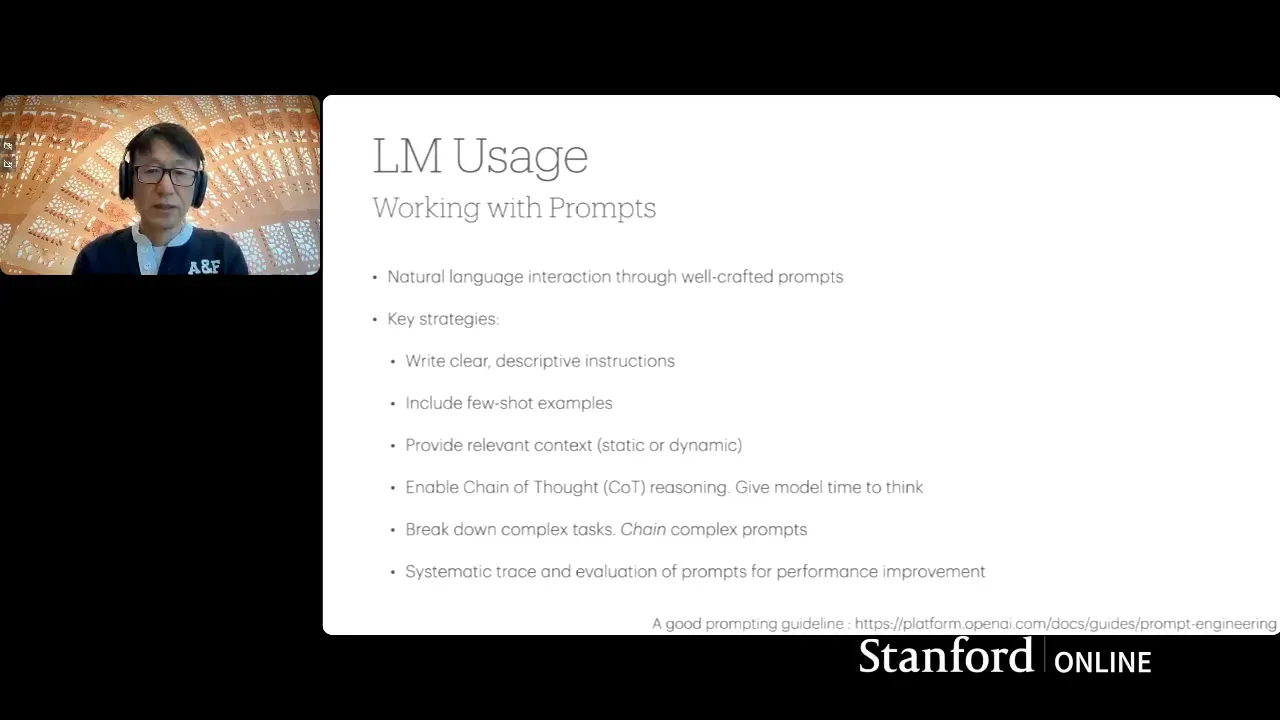
Examples of using Chain of Thought to solve problems
For example, when the model is given to evaluate whether a student's answer is correct or not. Instead of having the model answer right away, you might let the model solve the problem and generate its own answer first. Then compare it with the students' answers. This allows the model to provide more accurate and logical answers.
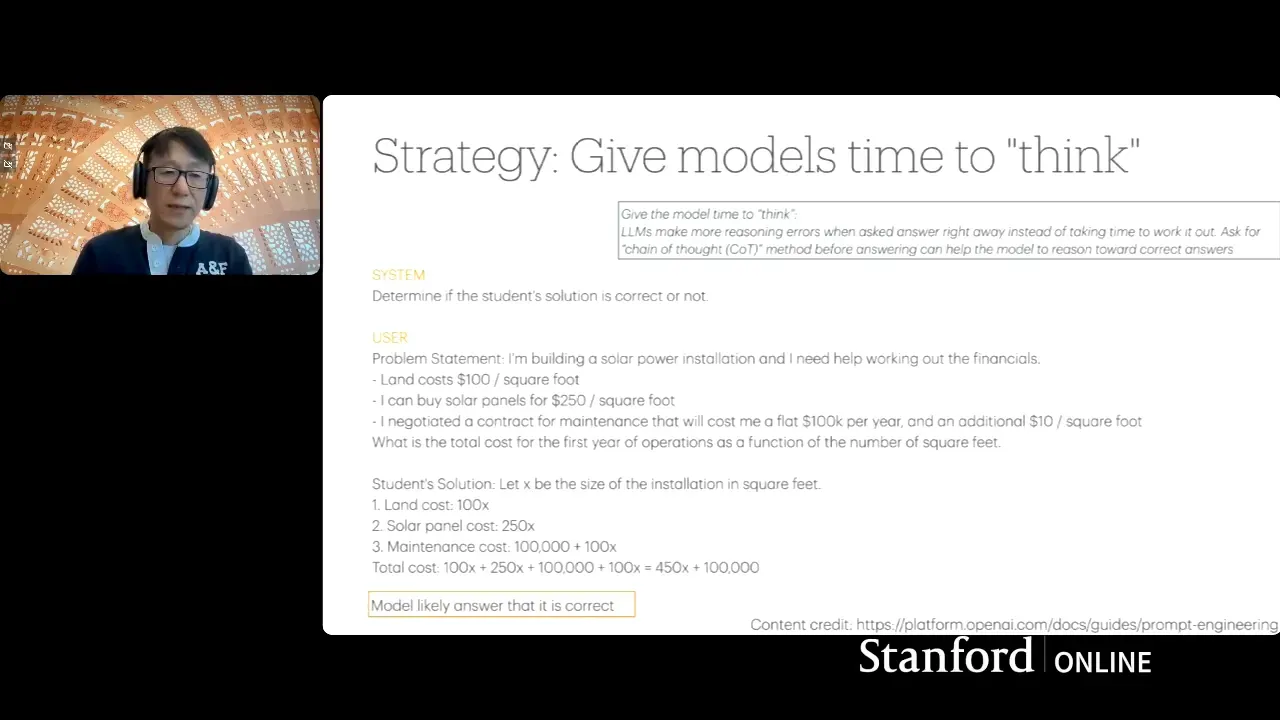
Limitations of language models and how to fix them
Although language models are highly effective, there are still limitations that need to be addressed, such as:
- Hallucination: The model may generate inaccurate or non-existent data. Especially in matters that require high accuracy, such as calculations or specialized data.
- Knowledge Cutoff: The model is trained with data over a period of time. This makes it impossible to know new information or the latest news that happens after that.
- Lack of Attribution: The model cannot tell where the generated data came from.
- Context Length Constraints: The model can perceive information in a limited context, which may not be enough for complex tasks.
One of the solutions is to use techniques. Retrieval Augmented Generation (RAG) This reduces the problem of generating data errors by using relevant information from databases or external sources to compile answers.
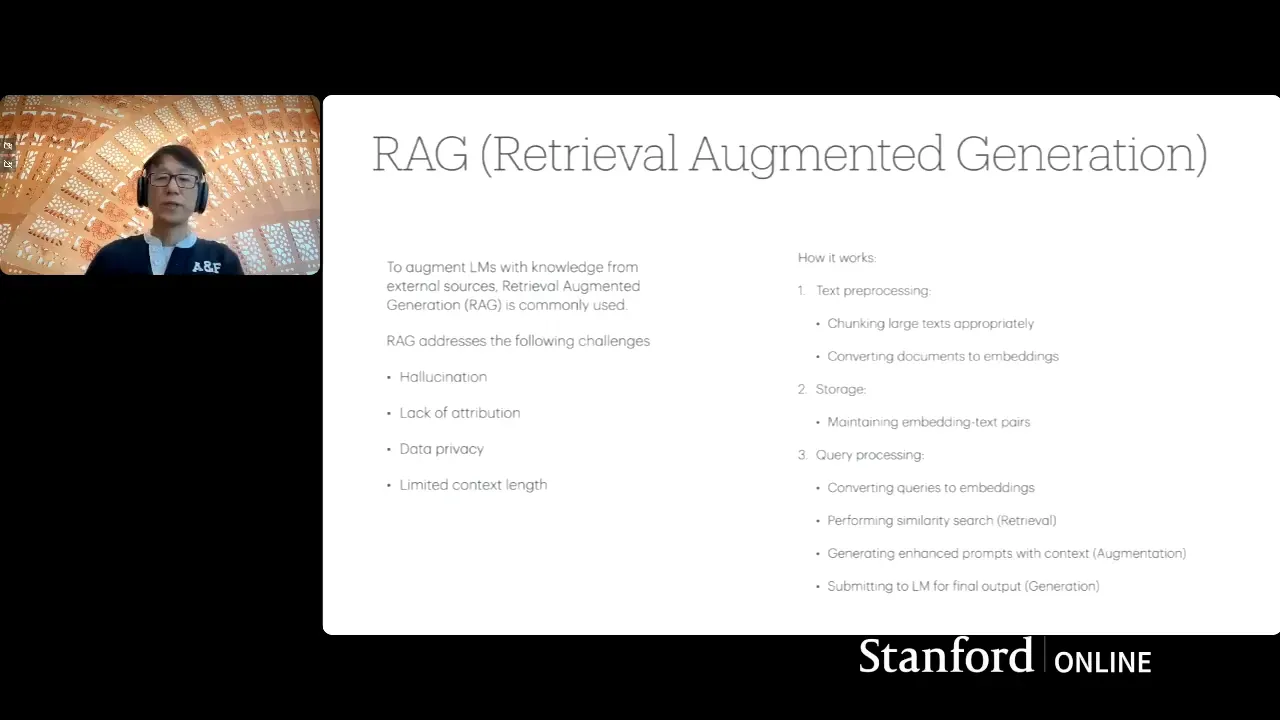
Tool Usage with Language Models
Language models usually only receive and send messages. However, increasing the ability of the model to use external tools such as calling API This allows the model to perform complex tasks and respond better to real-world situations.
For example, when a model is asked, "What is the weather like in San Francisco?" The model generates commands for external systems to call the API, retrieve the actual weather data, and then send the results back to the model so that the model generates appropriate and user-friendly responses.
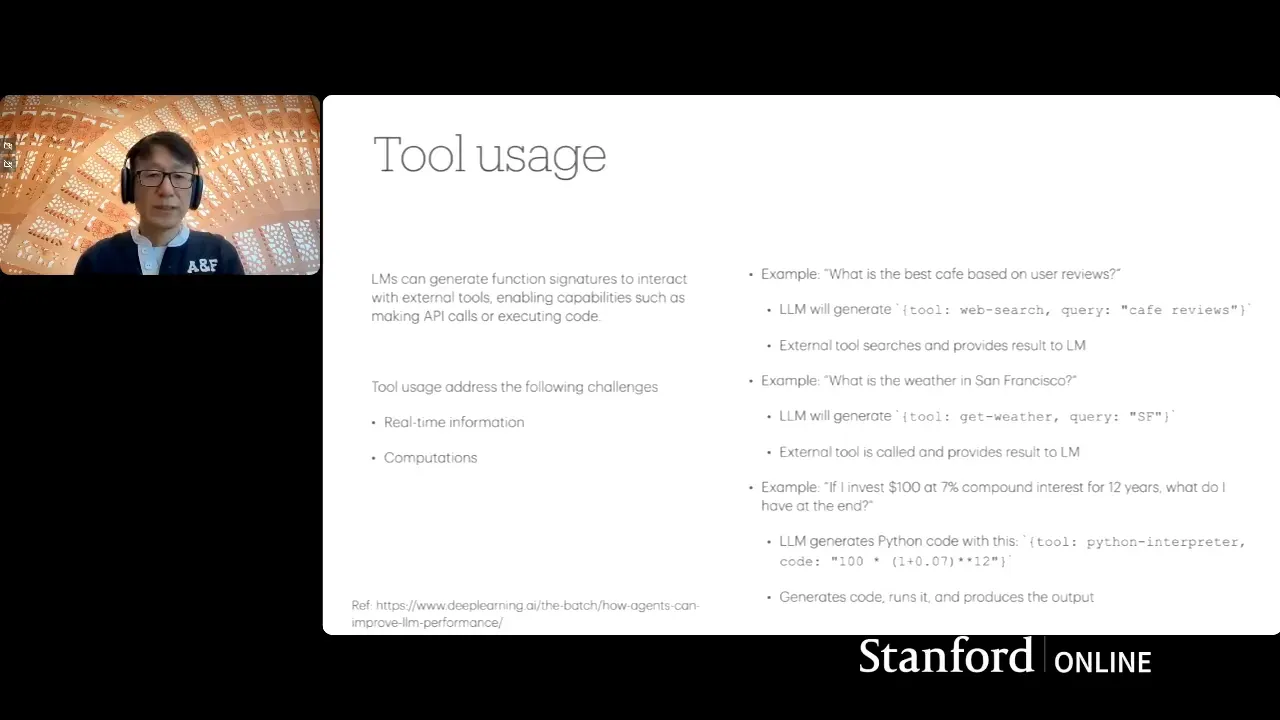
Agentic Language Model: A language model with the ability to interact with the environment.
Agentic Language Model It is the evolution of language models that not only receive and send messages, but can also interact with the external environment, such as using tools, searching for information, and storing data in memory to make decisions and take continuous action.
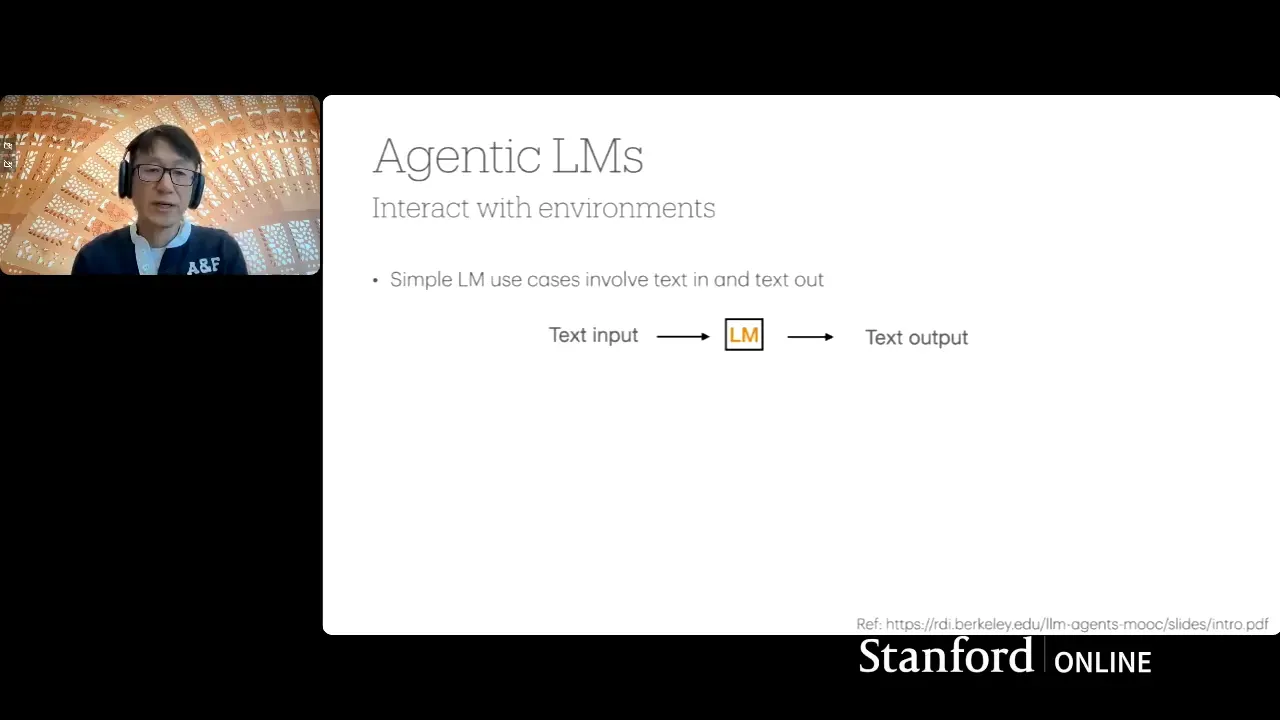
Agentic Language Model It consists of two main parts:
- Reasoning: The model can plan and analyze problems in a stepwise manner, such as breaking down complex tasks into smaller tasks to make them easier to manage.
- Action: Models can run tools or APIs to retrieve data or perform real-world tasks, such as retrieving data from a database or executing code to validate the results.
Examples of using the Agentic Language Model in customer service
Let's say a customer asks, "Can I get a refund for this item?" system Agentic AI It works by dividing the question into sub-steps, such as:
- Check the company's refund policy.
- Review customer information
- Check the information of the products ordered by the customer.
- Summarize and decide whether to allow a refund or not.
At each stage, the model runs an API or external database system to retrieve the necessary data, then processes all the data and generates the right answer for the customer.
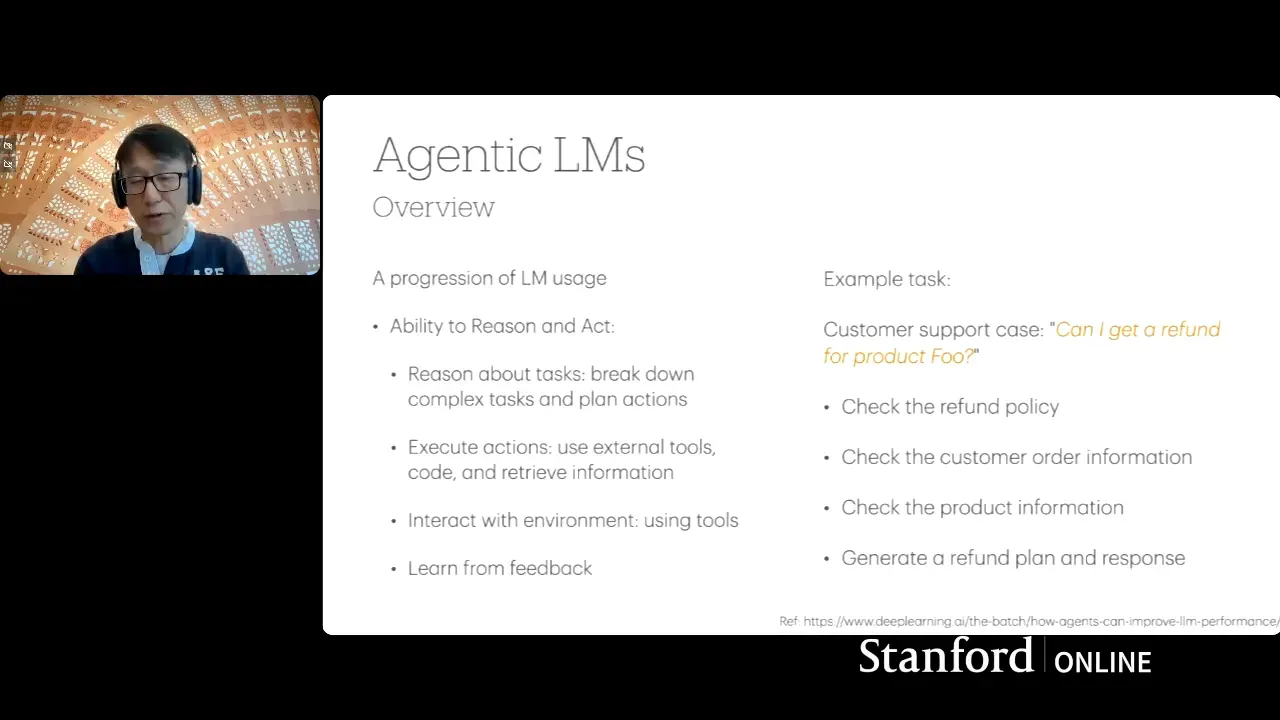
Design and Implementation of the Agentic Language Model
design Agentic AI It has a variety of variations, which allows the model to work more complex and efficiently. The main guidelines are as follows:
1. Planning
Let the model help plan and divide complex tasks into clear subtasks to ensure systematic and efficient execution.
2. Reflection
Use the model to evaluate and critique the results generated by the model itself to improve and increase accuracy, such as having the model review and modify the code before delivery.
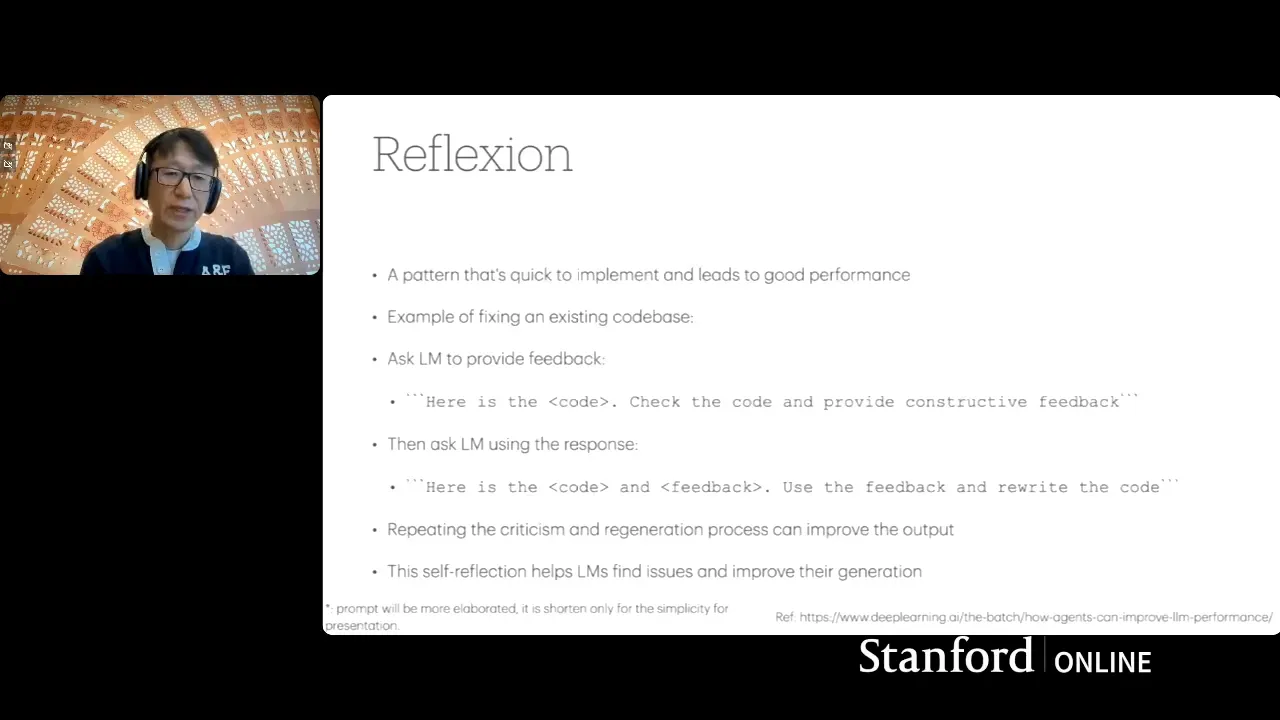
3. Tool Usage
Allow models to run external tools, such as APIs, or run code in sandboxes to help achieve more accurate and responsive results.
4. Multi-Agent Collaboration
Divide the work into small pieces and assign each agent a specific function, such as climate controllers, smart home lighting controllers, and use a central system to coordinate between these agents.
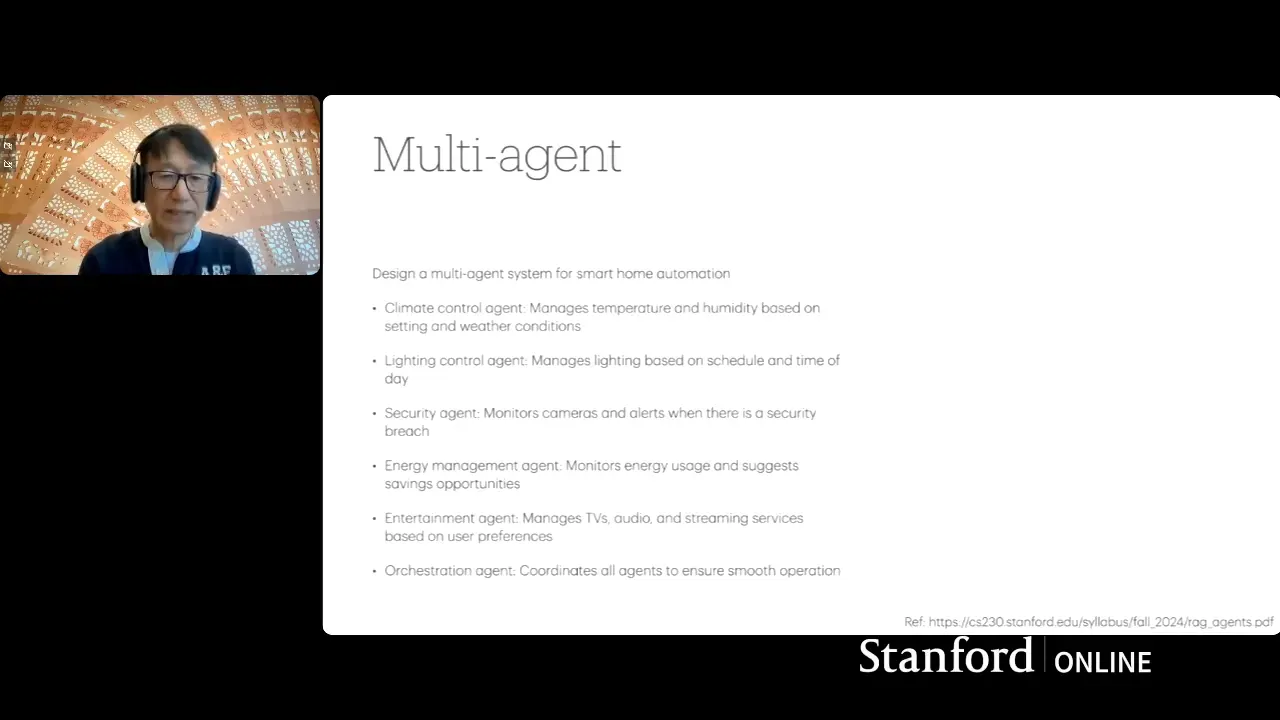
Practical Applications of Agentic AI
Agentic AI is used in a variety of fields, such as:
- Software development and editing, such as automated code generation or bug fixing.
- Data research and analysis, such as collecting data and summarizing results for users.
- Automation in organizations, such as customer service automation or office assistants.
Evaluation and Precautions for Using Agentic AI
Agentic AI evaluation is more complex than evaluating a simple language model because it requires consideration of the functionality of multiple agents and their interactions with each other.
One effective way is to use another language model that acts as an evaluator ( LLM as Judge ) may use the Reflection To make the assessment more detailed and reliable.
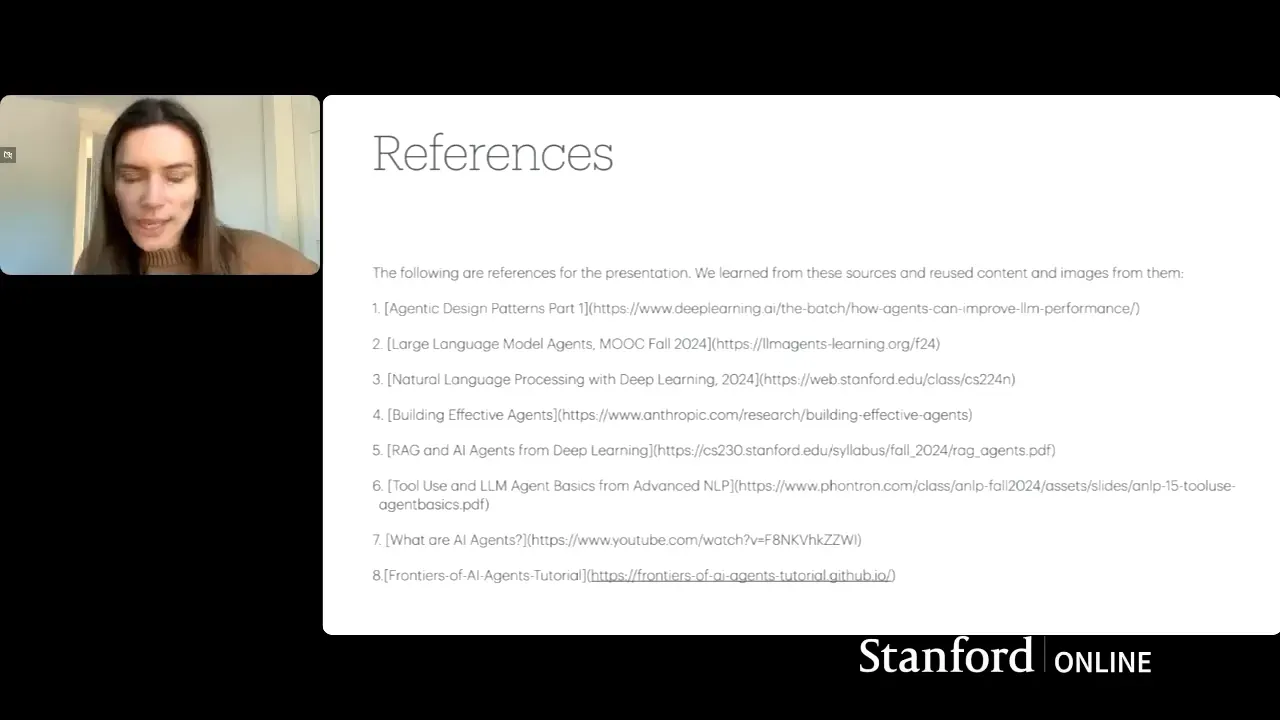
In addition, criteria and monitoring systems should be established to prevent ethical problems such as the creation of false information or inappropriate content ( Hallucination You may use a small model or a classifier to check the results before using them.
How to get started with Agentic AI?
The recommendation is to start by using the simplified language model first in the Playground of the model provider to experiment with creating a prompt and see the results, and then moving to use the API in the development of the actual application.
Once you understand the basics, you can experiment with the Agentic Language Model features and existing frameworks to create a more complex and responsive system.
Resources and Keeping Up with AI News
Because the AI industry and language models are changing rapidly. Tracking news And new research from experts through channels such as Twitter, YouTube, and online courses is important.
It is important to find reliable sources that are appropriate for your level of knowledge so that you can constantly update new techniques and guidelines.
Technical Glossary
- Language Model: An AI model that is trained to predict the next word in a text message.
- Pre-Training: The process of training a model with a large amount of data to learn a language in general.
- Post-Training: The process of customizing the model to better respond to commands and questions.
- Prompting: Preparing a command text or question to send to a language model.
- Chain of Thought (COT): The technique is to make the model think in steps before giving an answer.
- Hallucination: The problem of generating false or untrue data by the model
- Retrieval Augmented Generation (RAG): Techniques to extract information from external sources to help create answers.
- Agentic Language Model: Language models that can interact with different environments and tools.
- Reflection: Letting the model evaluate and critique its own results to improve it.
- Multi-Agent Collaboration: A system that uses multiple agents working together to solve complex problems.
Conclusion from Insiderly
Agentic AI is an important step in developing language models that can work intelligently and flexibly with the outside world. By incorporating techniques such as planning, reflection, and the use of complementary tools, AI can solve complex problems and meet user needs much better.
For those interested in getting started, it's a good idea to experiment with language models at a basic level first, and then expand to Agentic AI using existing tools and frameworks.
Evaluation and ethical criteria should not be overlooked because data reliability and security are at the heart of the widespread use of AI.